16 JUNE 2020
Apollo 1060 - A New Paradigm in Chemical Space Exploration
Our team has developed Apollo 1060, a patent-pending AI-augmented decision-making platform for small molecule drug discovery that can rapidly search a chemical space that is orders of magnitude larger than existing methods. Our purpose at 99andBeyond is to develop and provide access to transformative new precision medicines to extend the healthspan of one billion people by 2050.
In collaboration with MIT and Mila researchers including a Turing laureate, we recently published at ICML about the methodology behind Apollo 1060 which describes a new paradigm in chemical space exploration that can rapidly search an estimated 1025 readily-producible small molecules with 5 or fewer chemical reactions[1] – more than the number of stars in the universe. Our approach is unique to ensure that the AI-generated candidate drugs can be produced in a laboratory and a turnaround time for production in a matter of weeks rather than months. Moreover, the properties of the molecules we generate are more desirable than existing state-of-the-art algorithms using common benchmarks and a simulated proof-of-concept that mimics the drug discovery process. Ultimately, our recently published research lays the foundation for efficiently using AI to rapidly search the chemical space which comprises an abundance of miracle medicines.
Why Chemical Space Exploration?
The chemical space encompassing all drug-like molecules is immense in size with an estimated 1060 small molecules – more than all the atoms in the solar system. Drug discovery involves searching this chemical space for drug-like molecules that satisfy multiple properties such as biological activity, safety and bioavailability simultaneously akin to searching for a needle in the haystack. Traditionally, chemists have relied on chemical intuition, virtual screening, and serendipity to dream up such new molecules. However, the mind-boggling size of the chemical universe makes it extremely hard to design novel molecules that satisfy all the desired parameters. Chemists must also determine the necessary chemical synthesis to make those molecules, which is currently treated as a separate step from identifying which molecules to make and contributes to the high labor and experimental costs of drug discovery. The design of novel drugs is a slow and laborious process that can involve several years of efforts with many failed attempts - an average time for pre-clinical research of 31 months [2] and out-of-pocket and capitalized costs, respectively, of $430 million and $1,098 million per approved new drug. [3]
“ Enumeration from first principles shows that almost all small molecules (>99.9%) have never been synthesized and are still available to be prepared and tested.”
Towards the beginning of the 1970s, researchers in industry and academia began utilizing computational models to assist chemists in generating new molecules that exhibit desirable properties. These models have enabled the enumeration of large chemical databases that can comprise up to trillions of molecules which are used in the search for potential drugs. For example, the Enamine Real Library is limited to 13 billion (1010) molecules [5], the Pfizer Global Virtual Library enumerates 10 trillion (1013) compounds [6], Evotec’s EVOspace counts a little bit more than 10 quadrillion (1016) molecules [7], AstraZeneca’s Space covers 100 quadrillion (1017) compounds [8] and the Merck MASSIV Library comprises 100 quintillion (1020) molecules [9], corresponding to a drop in the ocean relative to the small molecule chemical space. In fact, the scalability of such libraries is limited because it would be impossible to enumerate every possible molecule.
“ But the real potential in AI algorithms comes from exploring larger chemical spaces for de novo designs. ”
In the past decade, AI has gone from a niche research topic in academic labs towards the mainstream. There has been significant progress in AI for small molecule drug discovery, particularly in generative modeling of molecular structures using approaches such as variational auto-encoders [11-12], generative adversarial networks [13-15] and reinforcement learning [16-19]. However, current approaches including evolutionary algorithms exhibit a significant challenge as they do not ensure that the proposed molecular structures can be produced, thereby seriously limiting their practical applicability. [20] Put simply, traditional generative models generate chemical structures without considering how hard it will be to make them.
“ Most AI approaches to drug discovery focus on answering the question of what compounds to make, but miss how to make those compounds. ”
How is Apollo 1060 Different?
To navigate the chemical space efficiently, we propose an approach that considers a candidate drug as a sequence of chemical transformations applied to an initial molecule. Apollo 1060 is based on research we recently published at ICML that utilizes reinforcement learning, a subset of machine learning where software agents takes actions in an environment to maximize a given reward; in our case, to learn to select the best set of commercially available reagents and valid chemical transformations that maximize the desired properties of the product molecule. This guarantees that each molecule proposed by the system can be produced and also provides the corresponding reactions for production.
Organization | Name | Number of Compounds | Reference |
---|---|---|---|
99andBeyond | Apollo 1060 | 1E+25 | 1 |
Merck KGaA | MASSIV | 1E+20 | 8 |
AstraZeneca | AZ Space | 1E+17 | 7 |
Evotec | EVOspace | 1.6E+16 | 6 |
Pfizer | PGVL | 3E+12 | 5 |
Boehringer Ingelheim | BICLAIM | 5E+11 | 22 |
Eli Lilly | Lilly LPC | 2E+11 | 23 |
University of Bern | GDB-17 | 2E+11 | 3 |
Enamine | Real | 13,000,000,000 | 4 |
NCI | SAVI | 1,750,000,000 | 24 |
UCSF | ZINC15 | 750,000,000 | 25 |
NCBI | PubChem Compounds | 102,710,073 | 26 |
University of Dortmund | CHIPMUNK | 100,000,000 | 27 |
Wuxi Apptec | Wuxi Virtual | 100,000,000 | 28 |
University of Marburg | SCUBIDOO | 21,000,000 | 29 |
Table 1: Large virtual chemical spaces for small molecule drug design (Switch to computer to see table)
In theory, our approach can rapidly search an estimated 10 25 readily-producible small molecules with 5 or fewer chemical reactions. In comparison, it is estimated that there are approximately 10 24 stars in the universe according to the European Space Agency. The algorithm we propose doesn’t involve an exhaustive search of a database and instead learns to efficiently search through the chemical space. Apollo 1060 achieves state-of-the-art performance across the most widely used benchmarks in generative modeling of chemical structures including QED, the quantitative estimate of drug-likeness, and penalized ClogP, an estimate of the octanol-water partition coefficient. Moreover, in an in silico proof-of-concept utilizing a predictive model of activity against three HIV targets, our approach outperformed competing AI approaches, demonstrating the power of our approach in optimizing for predicted biological activity.
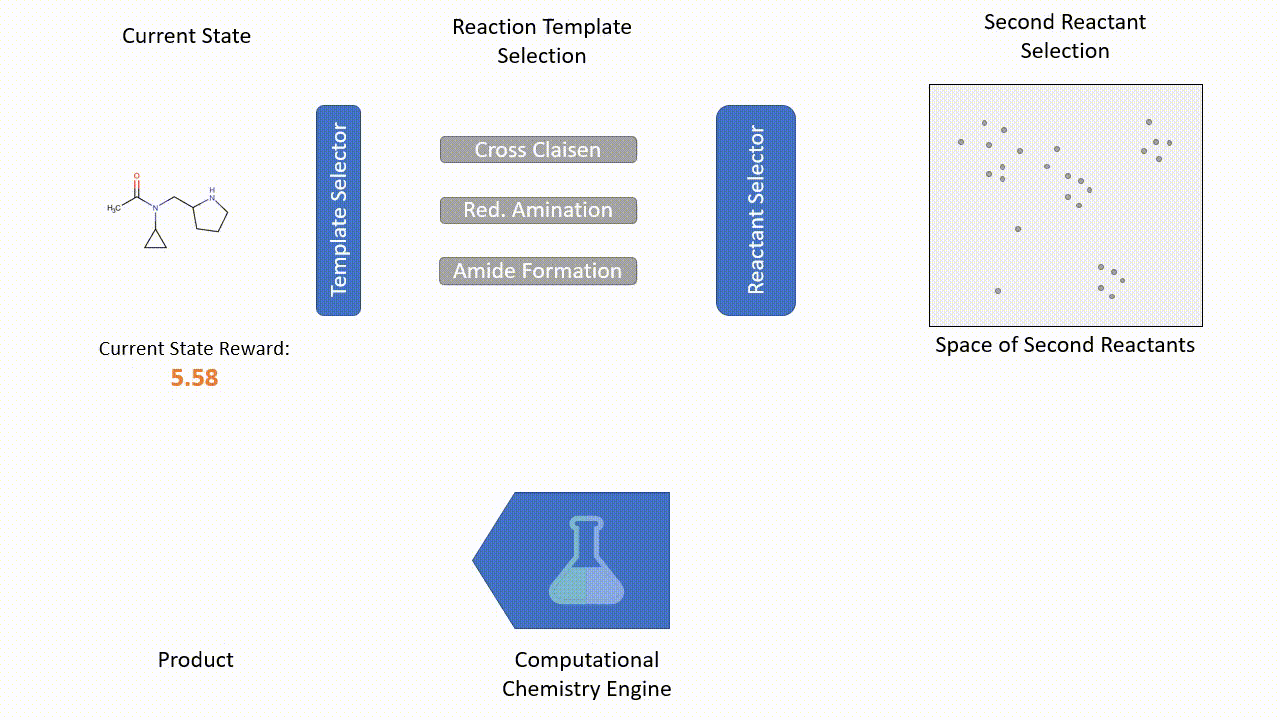
What Comes Next?
The current ligand-based technology increases the size of the chemical space and provides a faster turnaround time for production, however, predictive models for biological activity require a lot of experimental data to achieve good accuracy. To address this, we plan on integrating the latest experimental and computational techniques that consider the interaction between the molecule and the three-dimensional structure of the biological target determined through x-ray crystallography or cryo-electron microscopy.
“ There’s an ongoing holy grail: Can you simulate and predict molecular movement and molecular dynamics? ”
We’re building 99andBeyond as a full-stack AI-driven pharmaceutical company and are assembling a highly interdisciplinary team that brings together experts in cheminformatics, drug discovery and machine learning. We aim to leverage Apollo 1060 to bring transformative new precise treatments from conception to clinical testing in as little as 12 months, providing a much cheaper and faster alternative to the existing paradigm. Moreover, Apollo 1060 is target-agnostic and can be leveraged across any disease.
We’ve hardly scratched the surface of what’s possible. Increasing the number of consecutive reactions beyond five and utilizing a larger set of reaction templates and commercially available building blocks would exponentially increase the size of accessible chemical space beyond 1025, and ultimately unlock new accessible chemical space that could house an abundance of miracle medicines.